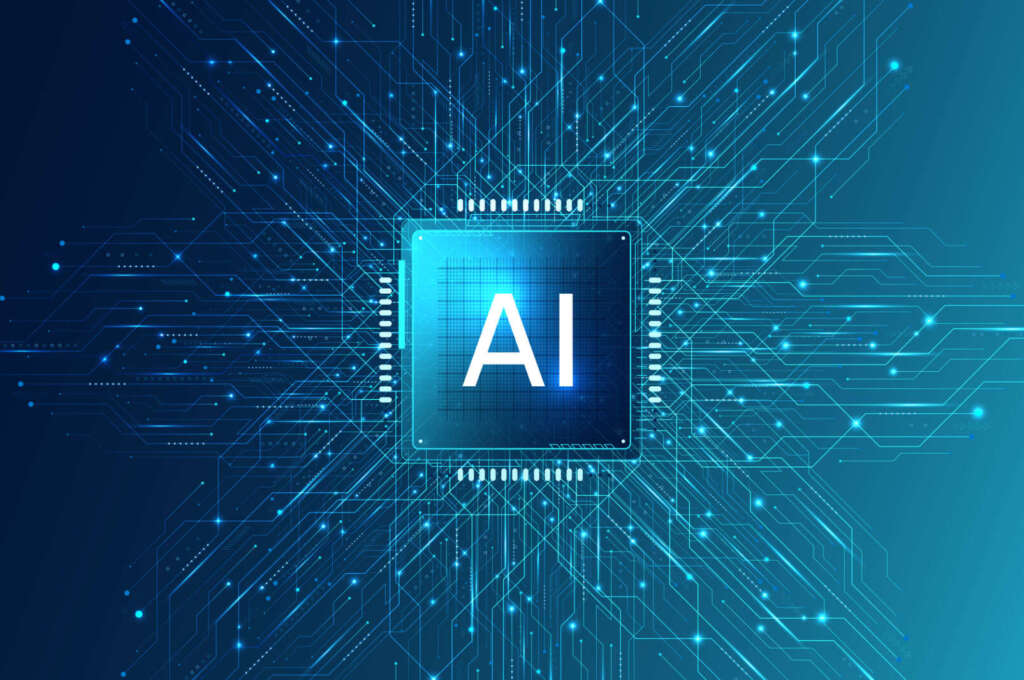
As artificial intelligence continues to reshape government operations, a new term is gaining traction among government AI leaders and AI development teams: “synthetic data.” However, a 2024 survey from Coleman Parkes and data and AI company SAS revealed that 32% of government decision-makers worldwide said they would not consider using synthetic data — significantly more than the 23% reported across general industries. This reluctance highlights a concerning gap in readiness, as public sector agencies risk falling behind in leveraging AI’s transformative potential.
How synthetic data is created and used
The term “synthetic data” refers to artificially-generated data that mimics the patterns and properties of real-world datasets. Created using advanced AI techniques like machine learning, synthetic data complements real-world data by filling gaps, enhancing analysis and enabling deeper insights. In the public sector, synthetic data is particularly valuable for training and testing AI systems or simulating scenarios without exposing sensitive or restricted information. Its core advantage lies in its ability to generalize and anonymize citizen data, allowing government agencies to make analyses and results publicly available while preserving individual privacy.
By understanding how synthetic data creates more representative datasets and exploring its practical applications, government leaders can unlock new possibilities for AI tools and empower state and federal agencies to make smarter, data-driven decisions without compromising security or privacy.
How synthetic data addresses public sector generative AI limitations
Generative AI has immense potential to enhance citizen services and streamline operations in the public sector. However, government bodies face constraints that often limit the full deployment of these technologies. Synthetic data provides a powerful tool to overcome these challenges:
Data privacy and security concerns
Real-world citizen data is often rich in sensitive personal information, making it difficult to use for AI training under stringent privacy regulations. Agencies must ensure that synthetic data does not expose sensitive information or allow tracing back to real source data. Techniques such as differential privacy can add noise to the data during the training and generation process, making it nearly impossible to re-identify individuals. Additionally, robust security measures can protect synthetic data from unauthorized access to maintain data privacy and security.
Invalid or low-quality data
Fragmented or siloed datasets often lack the consistency and depth needed for effective AI analysis. Synthetic data fills these gaps by generating high-quality, comprehensive datasets that more accurately represent the statistical properties of the original data without compromising its integrity. This involves using visual and statistical evaluation metrics to assess the synthetic data’s quality.
Additionally, it’s essential to validate the synthetic data by comparing it with real data (distributions and relationships) to ensure it meets the desired criteria and serves its intended purpose. Synthetic data must look like real data; otherwise, it cannot be trusted. Trustworthy synthetic data helps public-sector agencies improve the quality of insights derived from AI tools, leading to better policies and decisions, and more efficient service delivery.
Biased data
Bias in synthetic data, just as in real data, can lead to inaccurate and unfair outcomes, especially in government machine learning models whose predictions are used to make decisions that impact citizens. It’s important to identify and mitigate any biases that may be present in the original data and ensure they are not amplified in the synthetic data. This involves analyzing the data for underrepresented segments or groups and purposely focusing the generation of synthetic data to balance the data distribution. Addressing biases will help in creating fair and unbiased synthetic data that can be used for reliable decision-making.
Synthetic data in action
Synthetic data isn’t a new concept — the Census Bureau introduced the Synthetic Longitudinal Business Database (SynLBD) in 2010 to provide researchers and policymakers with business data for analysis while safeguarding confidentiality. This early example demonstrated how synthetic data could bridge the gap between data privacy and utility, supporting insights into economic trends, industry shifts and employment changes.
What’s different today is the emergence of advanced data analysis and AI technologies. These tools allow us to generate synthetic data that is more accurate, representative and useful than ever before. Combined with AI-powered processes, they unlock the full potential of synthetic data, enabling deeper insights and more impactful research and innovation across industries.
In Europe, we have already seen synthetic data put to use in projects such as CitiVerse and CROWN, which use synthetic data to streamline smart city functionality and improve traffic conditions based on anonymized representations of driver behavior.
There is a lack of data for “black swan” events, such as public health emergencies or natural disasters, leaving AI systems ill-equipped to address these edge cases. Synthetic data can simulate such scenarios in controlled environments, training AI tools to predict, respond to and mitigate the impacts of these events. Smart cities are generating synthetic sensor data to help with flood mitigation and disaster planning. Synthetic data is also a boon to public health researchers, who are using it when real health data is scarce, such as with rare diseases or underrepresented populations.
The path forward for synthetic data in government
Synthetic data supports innovation by enabling secure, ethical experimentation while safeguarding citizen trust. The ability to generate scalable and diverse datasets ensures that public sector AI models are both robust and agile, helping government agencies deliver improved services and outcomes. By addressing these limitations, synthetic data empowers public sector leaders to embrace generative AI while maintaining compliance, fairness and public trust. It paves the way for more innovative, effective and citizen-focused solutions, enabling government agencies to fully realize AI’s transformative potential.
Harnessing the power of synthetic data requires a thoughtful approach to unlock its potential while mitigating risks. Poorly generated synthetic data can introduce bias or inaccuracies, undermining its utility and eroding trust in decision-making processes. Moreover, synthetic data is not inherently private or secure; without robust controls and rigorous testing, it can inadvertently expose sensitive information. To fully capitalize on its benefits, agencies must prioritize careful planning, thorough validation and the implementation of strict safeguards.
John Gottula is a principal advisor for AI and biostatistics in the public sector division for data and AI company, SAS. Also a professor at North Carolina A&T University, John’s technical interests include agriculture, composite AI and synthetic data.
The post AI’s new ally: Why synthetic data matters for government AI adoption first appeared on Federal News Network.